Uncertainty Quantification in Engineering Science
Costas Papadimitriou
The course deals with the management of uncertainties in engineering science. Various forms of uncertainty such as parametric uncertainty or measurement uncertainty are dealt using probability distributions. Probabilities are used as a means of quantification of uncertainty, and thus Probability Theory is used. Important theorems from Probability Theory are examined, with the most important being the Bayes Theorem. The Bayes Theorem is used to update our uncertainty about the parameters of a model using information contained in measured data of the real system that the model tries to describe. Several analytical and computational techniques are introduced to tackle the Bayesian computations, including Laplace asymptotic approximations and Stochastic Simulation Algorithms.
LessThe course deals with the management of uncertainties in engineering science. Various forms of uncertainty such as parametric uncertainty or measurement uncertainty are dealt using probability distributions. Probabilities are used as a means of quantification of uncertainty, and thus Probability Theory is used. Important theorems from Probability Theory are examined, with the most important being the Bayes Theorem. The Bayes Theorem is used to update our uncertainty about the parameters of a model using information contained in measured data of the real system that the model tries to describe. Several analytical and computational techniques are introduced to tackle the Bayesian computations, including Laplace asymptotic approximations and Stochastic Simulation Algorithms.
The course deals with the management of uncertainties in engineering science. Various forms of uncertainty such as parametric uncertainty or measurement uncertainty are dealt using probability distributions. Probabilities are used as a means of quantification of uncertainty, and thus Probability Theory is used. Important theorems from Probability Theory are examined, with the most important being the Bayes Theorem. The Bayes Theorem is used to update our uncertainty about the parameters of a model using information contained in measured data of the real system that the model tries to describe. Several analytical and computational techniques are introduced to tackle the Bayesian computations, including Laplace asymptotic approximations and Stochastic Simulation Algorithms.
Course Units
The Bayesian method of inference is introduced for estimating model parameters and their uncertainties. Bayesian inference is rooted in Bayes theorem which encapsulates our state of knowledge about a parameter using probability distributions, in light of information contained in measured data. Probability is interpreted as a measure of plausibility.
Using elements of Information Theory, the problem of designing experiments in an optimal way is considered. The goal is to find which experiment will yield the most informative data in order to make inference about model parameters. Information Entropy is used as a scalar measure of the uncertainty.
Sampling techniques are used to tackle the complex computations which arise in Bayesian calculations. These calculations involve probability integrals in high-dimensional spaces which cannot be solved analytically. Sampling methods are used to draw samples from probability distributions and use these samples to perform further important computations.
Open Academic Course
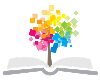
Num. of Visits : 1204
Num. of Hits : 13698
Calendar
Announcements
- - No existing announcements -